Preventing misinterpretation of cancer gene expression signatures
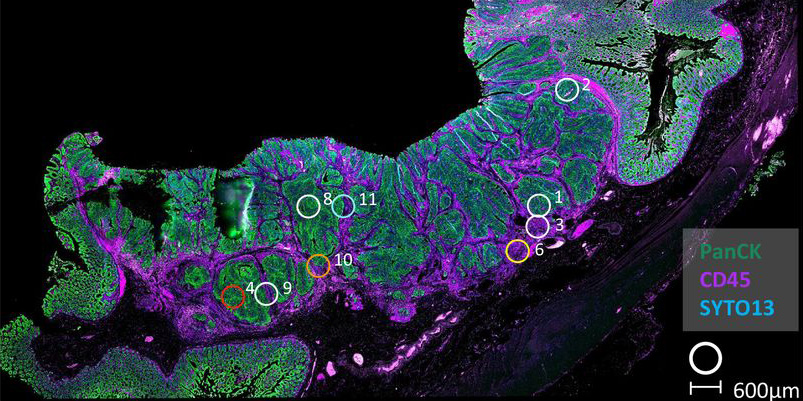
With so many different types (and sub-types) of cancer and a need for targeted therapies that will benefit any individual patient, gene expression signatures have become an important part of cancer research. They can be used as biomarkers for specific phenotypes or molecular mechanisms and to identify sub-types or suggest prognosis. However, a new study from Philip Dunne, a member of the MRC National Mouse Genetics Network’s Cancer cluster and a group leader at both the Patrick G. Johnston Centre for Cancer Research at Queen’s University Belfast and the Cancer Research UK Beatson Institute, has shown how gene signatures can be inadvertently misinterpreted, undermining their usefulness in distinguishing phenotypes in tumour samples.
Cancer is a complex disease or set of diseases. As well as differences associated with the parts of the body affected, differences in the underlying genetics can give very diverse outcomes. On top of that, a solid tumour is not a uniform lump of identical cancer cells, but is composed of a large variety of cell-types, many of which are not themselves cancerous, but might have been co-opted by the tumour or simply tricked to tolerate it. These non-cancer cells, known as the stroma, include connective tissue, vasculature, and immune cells.
Transcriptomics approaches that provide signatures for the gene expression in a cell or tissue have allowed scientists to sub-type cancers and link specific phenotypes to these biomarkers of functional activity. This addresses the genetic complexity of cancers as vast numbers of different signatures have been well-characterised to represent important cancer phenotypes. However, where these signatures have been characterised in clean in vitro models, there is a potential for misinterpretation when applying them to a complex tumour composed of variable amounts of different cell-types, as the stroma also contributes to the overall transcriptome of a tumour. Until now, there had been no detailed assessment of the consequences of stromal influence on the interpretation of gene expression signatures.
To address this problem, Philip’s group led a collaboration, with colleagues in Belfast, Glasgow, Oxford, and Zurich, including co-senior author Nigel Jamieson, Cancer cluster collaborator Simon Leedham, and Director of the Network, Owen Sansom, to perform a comprehensive assessment of approximately 8000 commonly employed transcriptional signatures in cancer research. Using various methodologies, including laser capture microdissection, flow cytometry, bulk and multiregional biopsy clinical samples, single cell RNA sequencing, and spatial transcriptomics, they were able to enumerate lineage-specific expression of each signature, meaning that they could see which components of the stroma might contribute to misinterpretations.
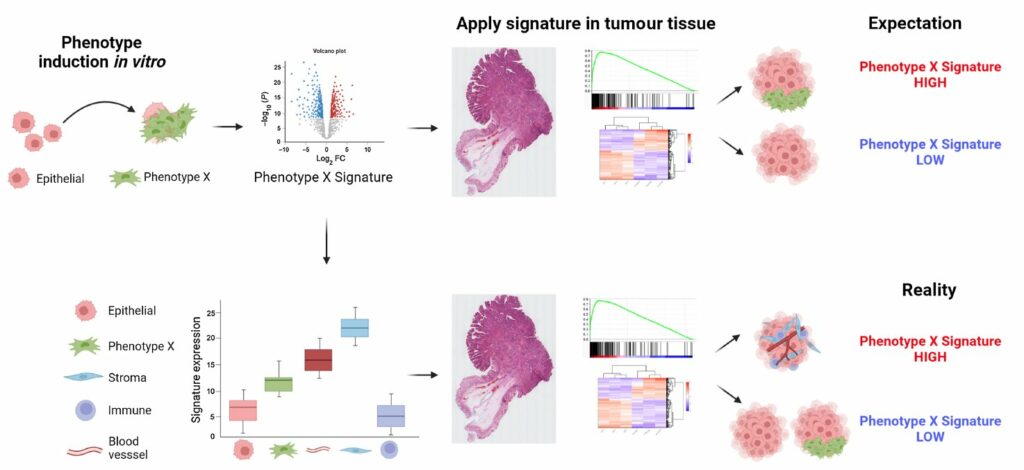
The results showed that, for some of the signatures, the stromal cells had expression orders of magnitude higher than the epithelial cells. When such results are applied to bulk tumour data, these signatures will simply measure the relative amount of stroma in the tumour, rather than provide an indication of the phenotype for which they were designed. Importantly, as well as confirming this finding, spatial transcriptomics also offered a solution, by demonstrating the importance of profiling samples with high resolution methods to ensure any interpretation of the gene signature is connected to an understanding of the tissue types present.
In addition, to ensure their findings can be widely applied, the team developed a freely available online resource, ConfoundR, which gives a user the ability to quickly and easily interrogate the potential confounding effects on any individual gene, combination of genes, and gene expression signature across colorectal, pancreatic, breast, ovarian, and prostate cancer datasets.
This study demonstrates the clear potential for misinterpretation of the meaning of gene expression signatures when applying them to complex clinical tumour samples. The aim of the National Mouse Genetics Network’s Cancer cluster is to develop the next generation of mouse models of cancer that better reflect specific subsets of human cancers by better matching the genetics and transcriptional phenotyping. These improved mouse models will only be possible with a precise understanding of the contributions of the stroma to the transcriptional readout and ensuring that the mice are developed to match the complex reality of human tumours.
Philip Dunne: “Integrity and robustness in aligning models with human tumours is critical in the era of precision medicine, where treatments are tailored to the biology underpinning specific cancer sub-types.”
This study was supported by UKRI MRC, Cancer Research UK, Fundación Científica de la Asociación Española Contra el Cáncer, Associazione Italiana per la Ricerca sul Cancro, and the Swiss National Science Foundation.
Fisher, NC, Byrne, RM, Leslie, H, Wood, C, Legrini, A, Cameron, AJ, Ahmaderaghi, B, Corry, SM, Malla, SB, Amirkhah, R, McCooey, AJ, Rogan, E, Redmond, KL, Sakhnevych, S, Domingo, E, Jackson, J, Loughrey, MB, Leedham, S, Maughan, T, Lawler, M, Sansom, OJ, Lamrock, F, Koelzer, VH, Jamieson, NB, Dunne, PD. Biological misinterpretation of transcriptional signatures in tumor samples can unknowingly undermine mechanistic understanding and faithful alignment with preclinical data. Clinical Cancer Research (2022) OF1-OF14 https://doi.org/10.1158/1078-0432.CCR-22-1102