Categorisation at the pathway level identifies a new cancer subtype
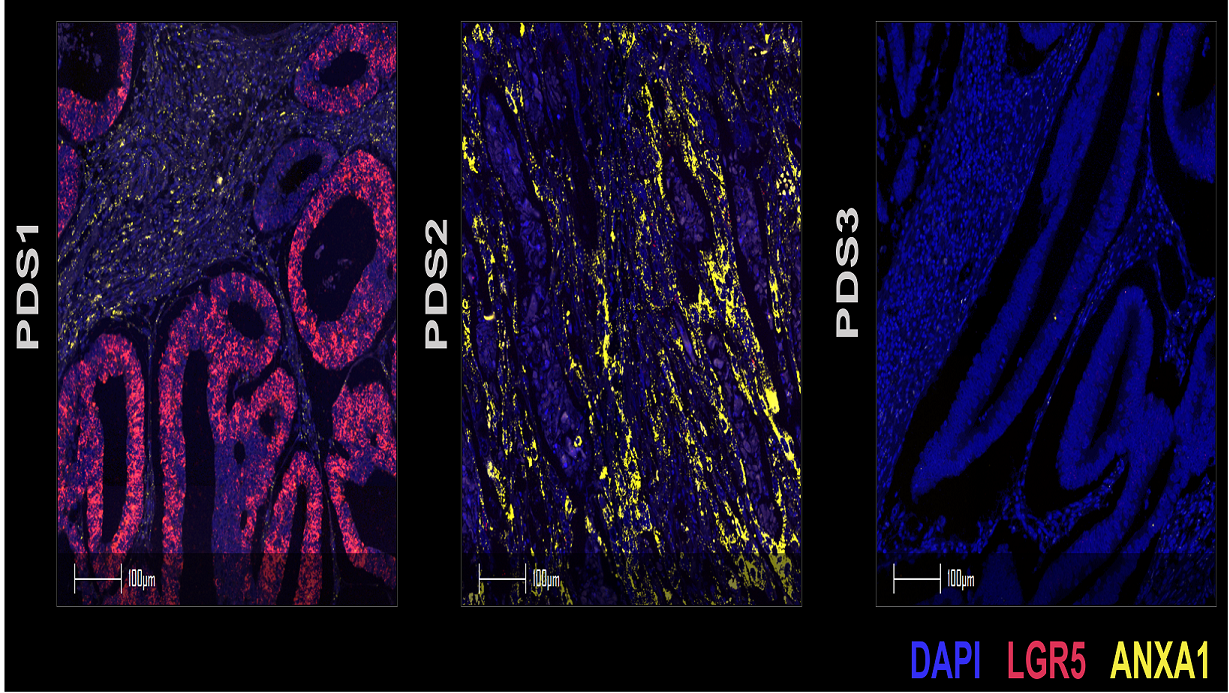
There are many different types and subtypes of cancer, and targeted therapies that will benefit an individual patient are much needed. Gene expression signatures have been routinely used to categorise individual tumours into various subtypes to suggest prognosis and treatment options. By instead making use of pathway-level data, Philip Dunne’s group and collaborators have identified a previously overlooked subtype of colorectal cancer that they found had a very poor prognosis.
Colorectal cancer can be classified into four consensus molecular subtypes (CMS1–4), based on their gene expression signatures. This subtype categorisation has prognostic value, but making comparisons with tumours in mouse models has been challenging as it requires mapping of human genes onto mouse equivalents, which isn’t always straightforward, due to possible differences in functionality. To address this, Philip’s group, who are members of the MRC National Mouse Genetics Network’s Cancer Cluster, developed a pathway-level approach to categorise colorectal cancers in mouse models for a more direct comparison between human and mouse data, enabling researchers to select the mouse models that are the best match for their tumour subtype of interest for preclinical testing.
In their new publication in Nature Genetics, led by Sudhir Malla, an ECR fellow in Philip’s group between the Patrick G. Johnston Centre for Cancer Research and CRUK Scotland Institute, the team describe how applying the same concept onto human colorectal cancers for classification led to them identifying three distinct pathway-derived subtypes (PDS1–3), with each accounting for roughly a third of colorectal cancers. Interestingly, this categorisation doesn’t correspond exactly with the CMS categories, as CMS2 tumours are distributed across PDS1 and PDS3, whereas CMS1 and CMS4 tumours are both within PDS2. In broad terms, PDS2 tumours were enriched for inflammatory and immune signalling pathways, PDS1 tumours displayed elevated cell-cycle-related pathways, and PDS3 unexpectedly showed near-universal repression of cancer-associated signalling, despite showing the worst prognosis in locally advanced disease.
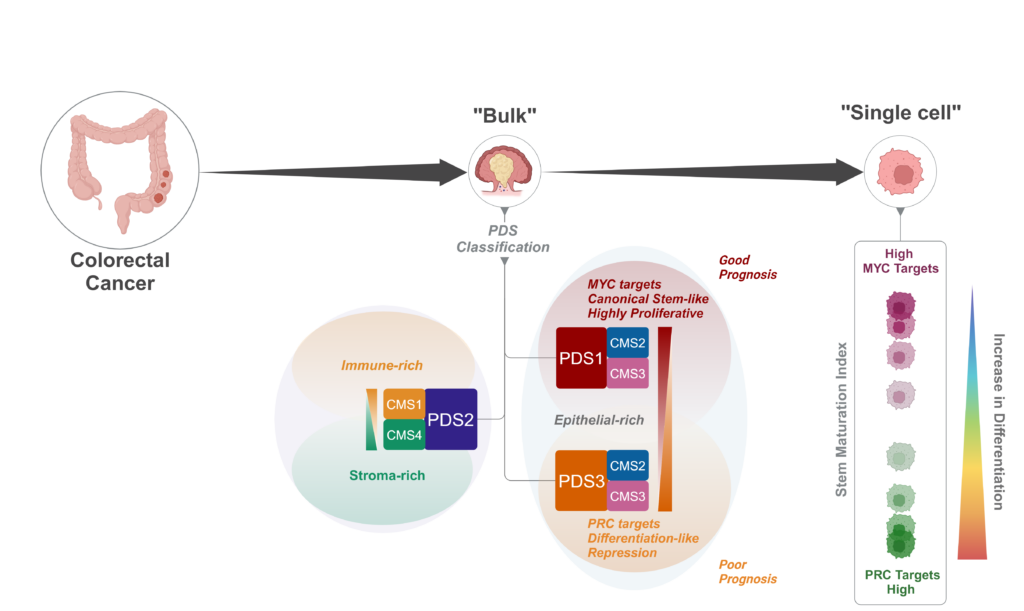
Despite having distinct biological signalling profiles, tumours in the different PDS groupings were mutationally indifferent and PDS3 tumours were morphologically indistinguishable from PDS1 and PDS2 tumours. One key difference found by the team was that PDS3 tumours were more differentiated-like along the cell lineage trajectory in contrast to the stem-associated, more highly proliferative PDS1 and PDS2 tumours.
When translating their PDS classification from human to a range of genetically altered mouse models and organoids, the team found that unlike PDS1 and PDS2 tumours, no model was found that faithfully recapitulates the biological signalling associated with human PDS3 tumours. This is likely explained by a bias towards developing models in which stem-rich tumours can be rapidly grown within the short lifespan of a mouse, which is completely unlike the slower-growing more differentiated PDS3 tumours identified in this study. The poor prognostic association of PDS3 highlights a clear requirement for new models to be developed in such a way that allows for the growth of tumours that better align with these more differentiated tumours.
This study demonstrates the benefit of using an approach to tumour classification that looks at transcriptomics data at a pathway level, rather than looking at individual gene clustering. This approach also prompted Philip’s group onto another research study, recently shared in bioRxiv where Sudhir co-supervised as senior author, which discusses the use of pairwise and single-sample pathway analytical tools and highlights how statistically significant differences from comparison between two groups-of-interest with a pairwise approach may be biologically misleading and may be indifferent when observed with a single-sample approach.
Sudhir Malla: “Uncovering a previously undetected unique tumour subset goes to show the value of a pathway-level single-sample approach in broad biological discovery, identifying a gap in the current representative tumour mouse models.”
The authors further emphasise that these different colorectal cancer classification systems should not be seen as competing, but complementary, and together will build up a more comprehensive understanding of tumours. This paper and their previous work to categorise colorectal tumours in mice highlight the value of the Network model to bring together expertise in mouse modelling with clinical data, as bioinformatics on human and mouse data points a way towards better mouse models that will enable the development of therapies for the cancer subtypes with the worst clinical outcomes.